- Item 1
- Item 2
- Item 3
- Item 4
Meta AI Unleashes Megabyte, a Revolutionary Scalable Model Architecture
Meta's research team unveils an innovative AI model architecture, capable of generating more than 1 million tokens across multiple formats and exceeding the capabilities of the existing Transformer architecture behind models like GPT-4.
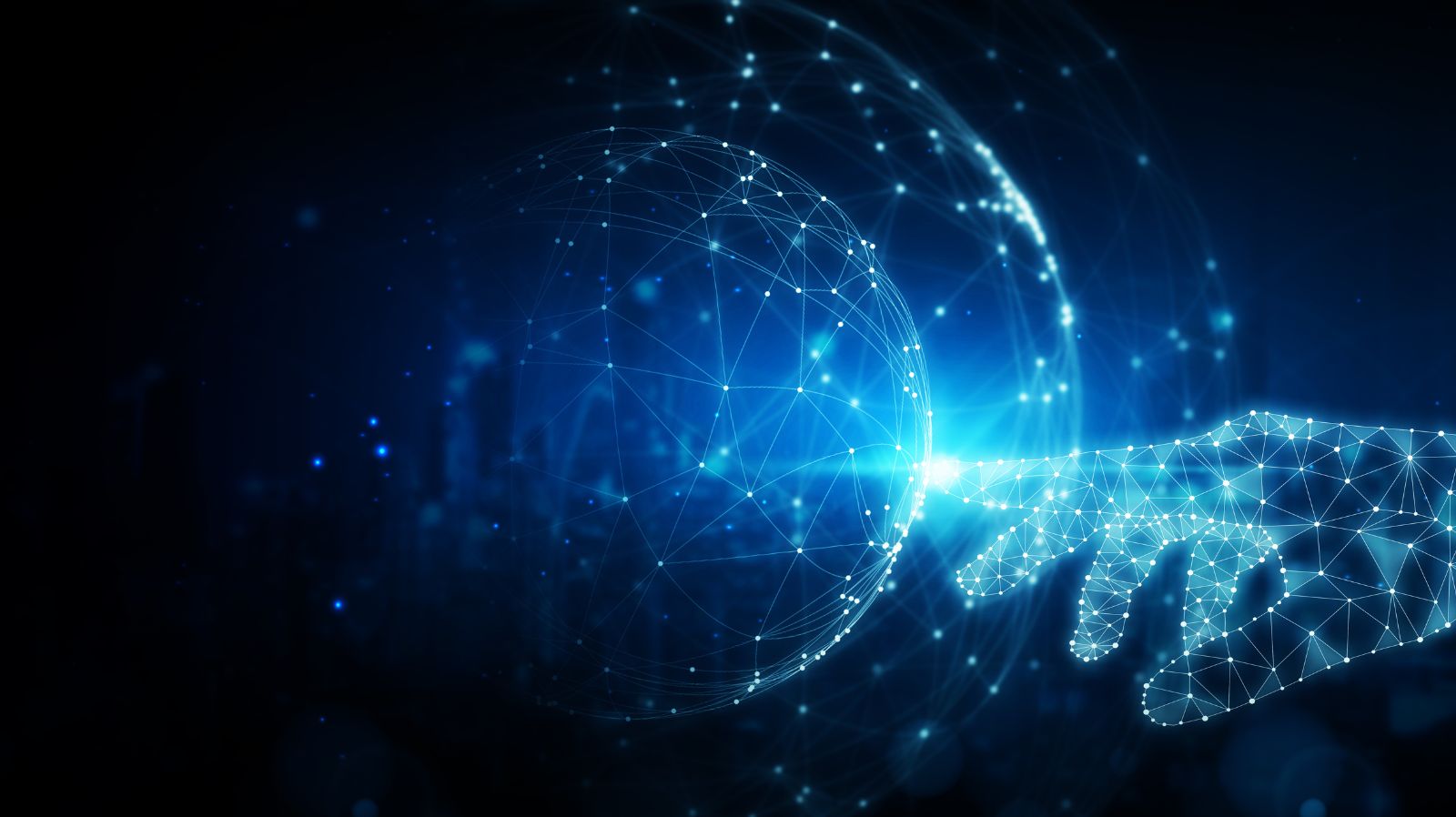
Meta's new proposed AI architecture could replace the popular Transformer models driving today's language models. Photo illustration: Artisana
đź§ Stay Ahead of the Curve
Meta AI researchers have proposed a groundbreaking architecture for AI decoder models, named the Megabyte model, capable of producing extensive content.
The Megabyte model addresses scalability issues in current models and performs calculations in parallel, boosting efficiency, and outperforming Transformers.
This innovation could instigate a new era in AI development, transcending the Transformer architecture and unlocking unprecedented capabilities in content generation.
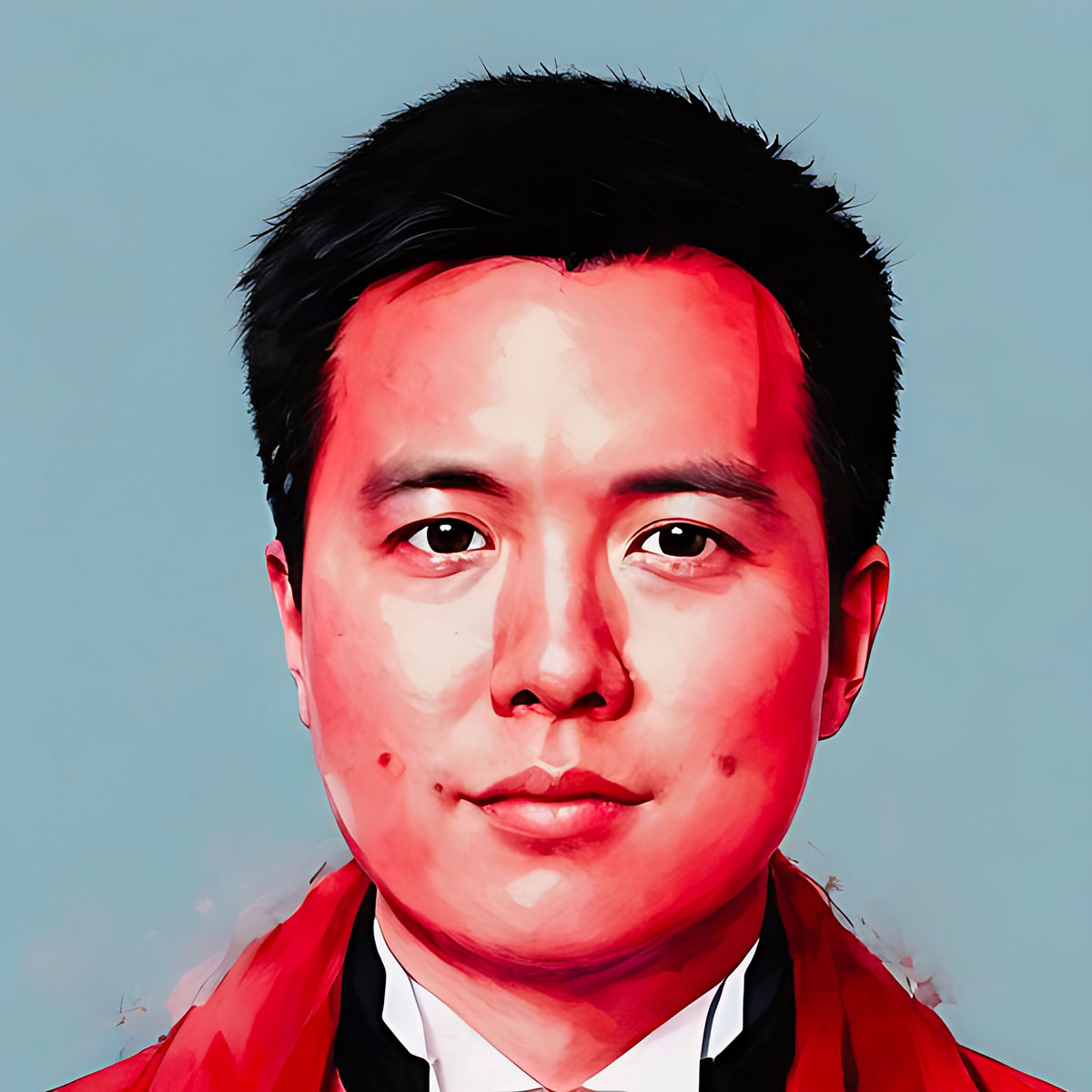
May 23, 2023
A Meta team of AI researchers has proposed an innovative architecture for AI models, capable of generating expansive content in text, image, and audio formats, stretching to over 1 million tokens. This groundbreaking proposal, if embraced, could pave the way for the next generation of proficient AI models, transcending the Transformer architecture that underpins models such as GPT-4 and Bard, and unleashing novel capacities in content generation.
The Constraints of Current Models
Contemporary high-performing generative AI models, like OpenAI's GPT-4, are grounded in the Transformer architecture. Initially introduced by Google researchers in 2017, this architecture forms the backbone of emergent AI models, facilitating an understanding of nuanced inputs and generating extensive sentences and documents.
Nonetheless, Meta's AI research team posits that the prevailing Transformer architecture might be reaching its threshold. They highlight two significant flaws inherent in the design:
With the increase in the length of inputs and outputs, self-attention scales dramatically. As each word processed or produced by a Transformer language model requires attention to all other words, the computation becomes highly intensive for thousands of words, whereas it's less problematic for smaller word counts.
Feedforward networks, which aid language models in comprehending and processing words through a sequence of mathematical operations and transformations, struggle with scalability on a per-position basis. These networks operate on character groups or "positions" independently, leading to substantial computational expenses.
Megabyte Model: The Game Changer
The Megabyte model, introduced by Meta AI, showcases a uniquely different architecture, dividing a sequence of inputs and outputs into "patches" rather than individual tokens. Within each patch, a local AI model generates results, while a global model manages and harmonizes the final output across all patches.
This methodology addresses the scalability challenges prevalent in today's AI models. The Megabyte model's patch system permits a single feedforward network to operate on a patch encompassing multiple tokens. Researchers found that this patch approach effectively counters the issue of self-attention scaling.
The patch model enables Megabyte to perform calculations in parallel, a stark contrast to traditional Transformers performing computations serially. Even when a base model has more parameters, this results in significant efficiencies. Experiments indicated that Megabyte, utilizing a 1.5B parameter model, could generate sequences 40% quicker than a Transformer model operating on 350M parameters.
Using several tests to determine the limits of this approach, researchers discovered that the Megabyte model's maximum capacity exceeded 1.2M tokens. For comparison, OpenAI's GPT-4 has a limit of 32,000 tokens, while Anthropic's Claude has a limit of 100,000 tokens.
Shaping the AI Future
As the AI arms race progresses, AI model enhancements largely stem from training on an ever-growing number of parameters, which are the values learned during an AI model's training phase. While GPT-3.5 was trained on 175B parameters, there's speculation that the more capable GPT-4 was trained on 1 trillion parameters.
OpenAI CEO Sam Altman recently suggested a shift in strategy, confirming that the company is thinking beyond training colossal models and is zeroing in on other optimizations. He equated the future of AI models to iPhone chips, where the majority of consumers are oblivious to the raw technical specifications. Altman envisioned a similar future for AI, emphasizing the continual increase in capability.
Meta’s researchers believe their innovative architecture arrives at an opportune time, but also acknowledge there are other pathways to optimization. Promising research areas such as more efficient encoder models adopting patching techniques, decode models breaking down sequences into smaller blocks, and preprocessing sequences into compressed tokens are on the horizon, and could extend the capabilities of the existing Transformer architecture for a new generation of models
Nonetheless, Meta’s recent research has AI experts excited. Andrej Karpathy, the former Sr. Director of AI at Tesla and now a lead AI engineer at OpenAI, chimed in as well on the paper. This is “promising,” he wrote on Twitter. “Everyone should hope that we can throw away tokenization in LLMs. Doing so naively creates (byte-level) sequences that are too long.”
Research
In Largest-Ever Turing Test, 1.5 Million Humans Guess Little Better Than ChanceJune 09, 2023
News
Leaked Google Memo Claiming “We Have No Moat, and Neither Does OpenAI” Shakes the AI WorldMay 05, 2023
Research
GPT AI Enables Scientists to Passively Decode Thoughts in Groundbreaking StudyMay 01, 2023
Research
GPT-4 Outperforms Elite Crowdworkers, Saving Researchers $500,000 and 20,000 hoursApril 11, 2023
Research
Generative Agents: Stanford's Groundbreaking AI Study Simulates Authentic Human BehaviorApril 10, 2023
Culture
As Online Users Increasingly Jailbreak ChatGPT in Creative Ways, Risks Abound for OpenAIMarch 27, 2023